Data Science Strategy For Dummies
ISBN: 9788126533367
364 pages
For more information write to us at: acadmktg@wiley.com
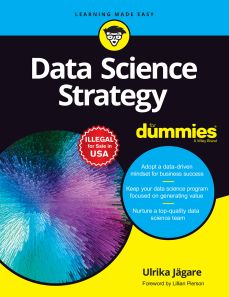
Description
Data Science Strategy For Dummies begins by explaining what exactly data science is and why it's important. While Using non-technical language, it covers mindsets, organizational players, processes and common roadblocks, all the while keeping a razor focus on business value and the nurturing of a top quality data science team.
Foreword
Introduction
About This Book
Foolish Assumptions
How This Book is Organized
Icons Used In This Book
Beyond The Book
Where To Go From Here
Part 1: Optimizing Your Data Science Investment
Chapter 1: Framing Data Science Strategy
- Establishing the Data Science Narrative
- Capture
- Maintain
- Process
- Analyze
- Communicate
- Actuate
- Sorting Out the Concept of a Data-driven Organization
- Approaching data-driven
- Being data obsessed
- Sorting Out the Concept of Machine Learning
- Defining and Scoping a Data Science Strategy
- Objectives
- Approach
- Choices
- Data
- Legal
- Ethics
- Competence
- Infrastructure
- Governance and security
- Commercial/business models
- Measurements
Chapter 2: Considering the Inherent Complexity in Data Science
- Diagnosing Complexity in Data Science
- Recognizing Complexity as a Potential
- Enrolling in Data Science Pitfalls 101
- Believing that all data is needed
- Thinking that investing in a data lake will solve all your problems
- Focusing on AI when analytics is enough
- Believing in the 1-tool approach
- Investing only in certain areas
- Leveraging the infrastructure for reporting rather than exploration
- Underestimating the need for skilled data scientists
- `Navigating the Complexity
Chapter 3: Dealing with Difficult Challenges
- Getting Data from There to Here
- Handling dependencies on data owned by others
- Managing data transfer and computation across-country borders
- Managing Data Consistency Across the Data Science Environment
- Securing Explain ability in AI
- Dealing with the Difference between Machine Learning and Traditional Software Programming
- Managing the Rapid AI Technology Evolution and Lack of Standardization
Chapter 4: Managing Change in Data Science
- Understanding Change Management in Data Science
- Approaching Change in Data Science
- Recognizing what to avoid when driving change in data science
- Using Data Science Techniques to Drive Successful Change
- Using digital engagement tools
- Applying social media analytics to identify stakeholder sentiment
- Capturing reference data in change projects
- Using data to select people for change roles
- Automating change metrics
- Getting Started
Part 2: Making Strategic Choices for Your Data
Chapter 5: Understanding the Past, Present